What is You Build It You Run It, and why does it have such a positive impact on operability? Why is it important to balance support cost effectiveness with operability incentives?
This is part of the Who Runs It series.
Introduction
The usual alternative to You Build It Ops Run It is for a Delivery team to assume responsibility for its Run activities, including deployments and production support. This is often referred to as You Build It You Run It.
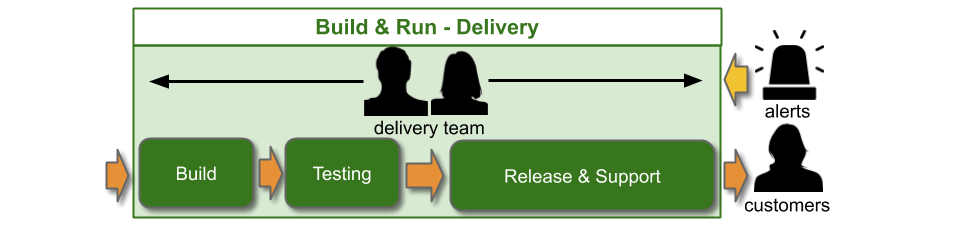
You Build It You Run It consists of single-level swarming support, with developers on-call. There is also a Service Desk to handle customer requests. The toolchain needs to include anomaly detection, alert notifications, messaging, and incident management tools, such as Prometheus, PagerDuty, Slack, and ServiceNow.
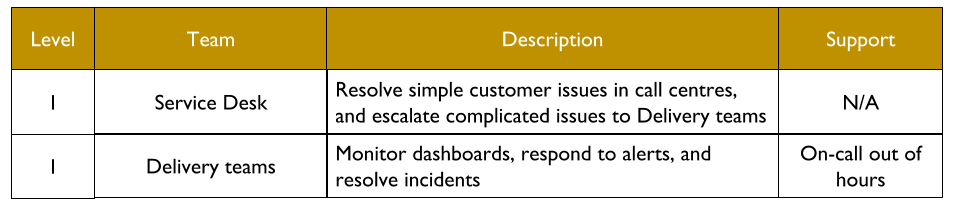
As with You Build It Ops Run It, Service Desk is an L1 team that receives customer requests and will resolve simple technology issues wherever possible. A development team in Delivery is also L1, and they will monitor dashboards, receive alerts, and respond to incidents. Service Desk should escalate tickets for particular website pages or user journeys into the incident management system, which would be linked to applications.
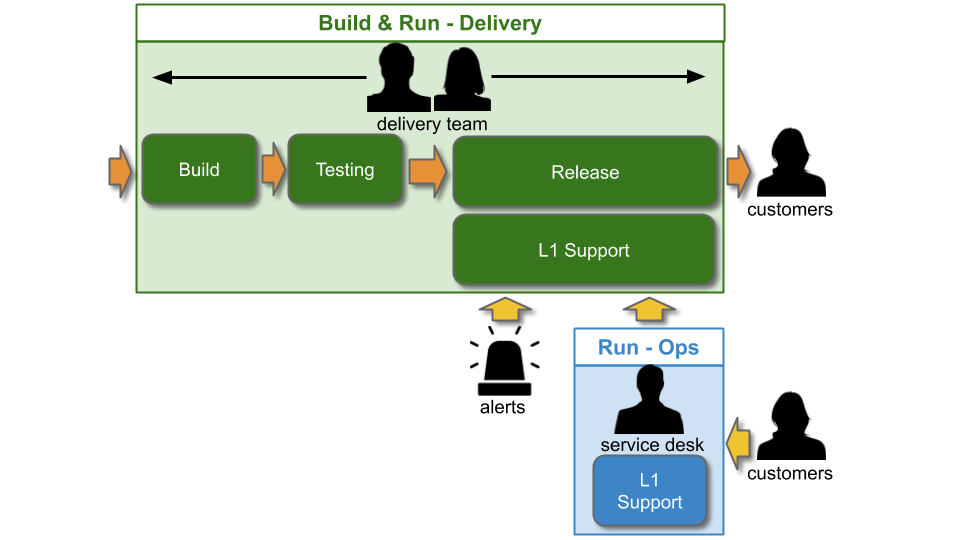
Delivery engineering costs and on-call support will both be paid out of CapEx, and Operations teams such as Service Desk will be under OpEx. As with You Build It Ops Run It, the Service Desk team might be outsourced to reduce OpEx costs. CapEx funding for You Build It You Run It will compel a product manager to balance their desired availability with on-call costs. OpEx funding for Delivery on-call should be avoided wherever possible, as it encourages product managers to artificially minimise risk tolerance and select high availability targets irregardless of on-call costs.
Continuous Delivery and operability
Swarming support means Delivery prioritising incident resolution over feature development, in line with the Continuous Delivery practice of Stop The Line and the Toyota Andon Cord. This encourages developers to limit failure blast radius wherever possible, and prevents them from deploying changes mid-incident that might exacerbate a failure. Swarming also increases learning, as it ensures developers are able to uncover perishable mid-incident information, and cross-pollinate their skills.
You Build It You Run It also has the following advantages for product development:
- Short deployment lead times – lead times will be minimised due to no handoffs
- Minimal knowledge synchronisation costs – developers will be able to easily share application and incident knowledge, to better prepare themselves for future incidents
- Focus on outcomes – teams will be empowered to deliver outcomes that test product hypotheses, and iterate based on user feedback
- Short incident resolution times – incident response will be quickened by no support ticket handoffs or rework
- Adaptive architecture – applications will be architected to limit failure blast radius, including bulkheads and circuit breakers
- Product telemetry – dashboards and alerts will be continually updated by developers, to be multi-level and tailored to the product context
- Traffic knowledge – an appreciation of the pitfalls and responsibilities inherent in managing live traffic will be factored into design work
- Rich situational awareness – developers will respond to incidents with the same context, ways of working, and tooling
- Clear on-call expectations – developers will be aware they are building applications they themselves will support, and they should be remunerated
You Build It You Run It creates the right incentives for operability. When Delivery is responsible for their own deployments and production support, product owners will be more aware of operational shortfalls, and pressed by developers to prioritise operational features alongside product ideas. Ensuring that application availability is the responsibility of everyone will improve outcomes and accelerate learning, particularly for developers who in IT As A Cost Centre are far removed from actual customers. Empowering delivery teams to do on-call 24×7 is the only way to maximise incentives to build operability in.
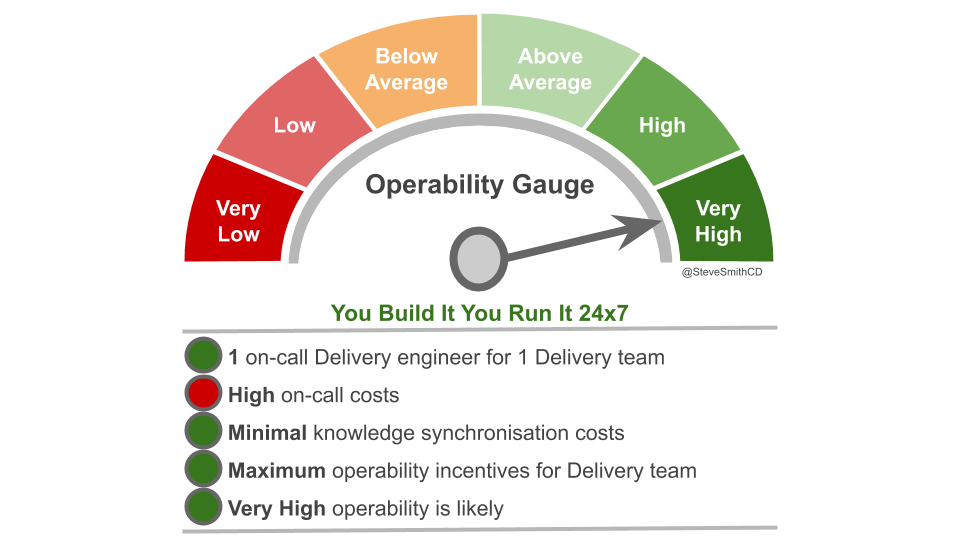
Production support as revenue insurance
The most common criticism of You Build It You Run It is that it is too expensive. Paying Delivery team members for L1 on-call standby and callout can seem costly, particularly when You Build It Ops Run It allows for L1-2 production support to be outsourced to cheaper third party suppliers. This perception should not be surprising, given David Wood’s assertion in The Flip Side Of Resilience that “graceful extensibility trades off with robust optimality”. Implementing You Build It You Run to increase adaptive capacity for future incidents may look wasteful, particularly if incidents are rare.
A more holistic perspective would be to treat production support as revenue insurance for availability targets, and consider risk in terms of revenue impact instead of incident count. A production support policy will cover:
- Availability protection
- Availability restoration on loss
You Build It You Run It maximises incentives for Delivery teams to focus from the outset on protecting availability, and it guarantees the callout of an L1 Delivery engineer to restore availability on loss. This should be demonstrable with a short Time To Restore (TTR), which could be measured via availability time series metrics or incident duration. That high level of risk coverage will come at a higher premium. This means You Build It You Run It will be more cost effective for applications with higher availability targets and greater potential for revenue loss.
You Build It Ops Run It offers a lower level of risk coverage at a lower premium, with weak incentives to protect application availability and an L2 Application Operations team to restore application availability. This will produce a higher TTR than You Build It You Run It. This may be acceptable for applications with lower availability targets and/or limited potential for revenue loss.
The cost effectiveness of a production support policy can be calculated per availability target by comparing its availability restoration capability with support cost. For example, at Fruits R Us there are 3 availability targets with estimated maximum revenue losses on availability target loss. Fruits R Us has a Delivery team with an on-call cost of £3K per calendar month and a TTR of 20 minutes, and an Application Operations team with a cost of £1.5K per month and a TTR of 1 hour.
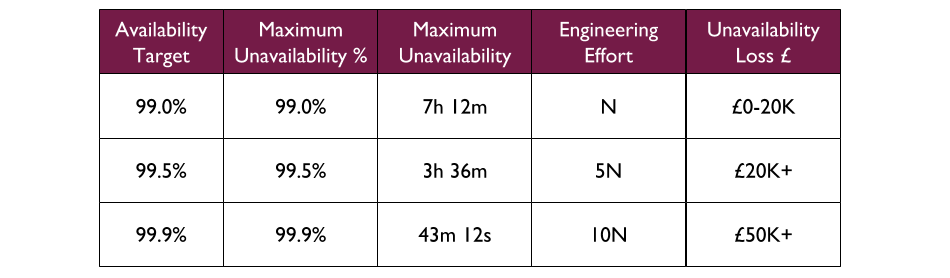
Projected availability loss per team is a function of TTR and the £ maximum availability loss per availability target, and lower losses can be calculated for the Delivery team due to its shorter TTR.
At 99.0%, Application Operations is as cost effective at availability restoration of a 7 hour 12 minute outage as the Delivery team, and Fruits R Us might consider the merits of You Build It Ops Run It. However, this would mean Application Operations would be unable to build operability in and increase availability protection, and the Delivery team would have few incentives to contribute.
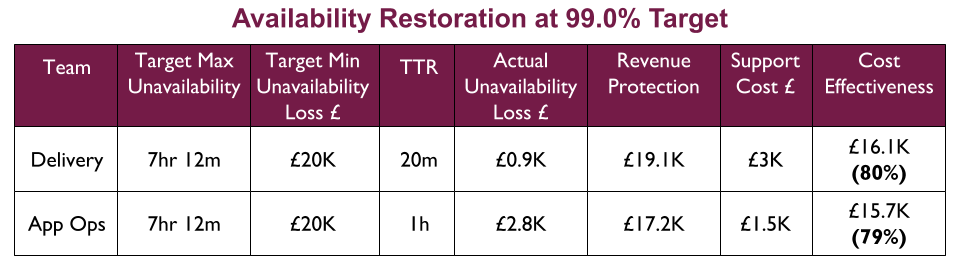
At 99.5%, the Delivery team is more cost effective at availability restoration of a 3 hour 36 minute outage than Application Operations.
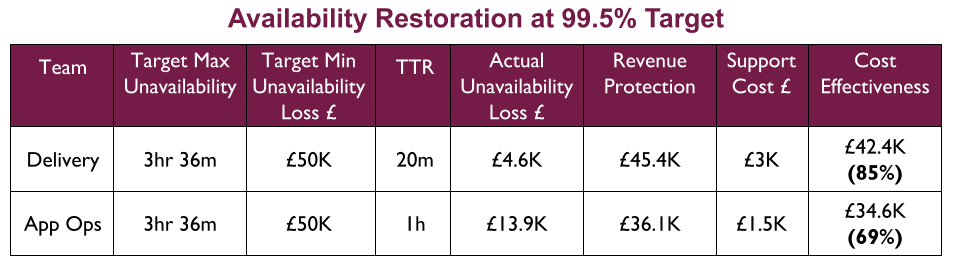
At 99.9%, the Delivery team is far more cost effective at availability restoration of a 43 minute 12 second outage. The 1 hour TTR of Application Operations means their £ projected availability loss is greater than the £ maximum availability loss at 99.9%. You Build It You Run It is the only choice.
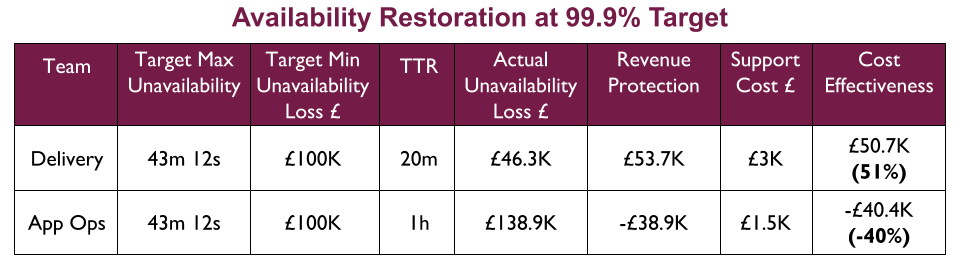
The Who Runs It series:
- You Build It Ops Run It
- You Build It You Run It
- You Build It Ops Run It at scale
- You Build It You Run It at scale
- You Build It Ops Sometimes Run It
- Implementing You Build It You Run It at scale
- You Build It SRE Run It
Acknowledgements
Thanks to Thierry de Pauw.